Image understanding
Deep learning techniques are the state-of-the-art in the field of Computer Vision. Deep neural networks are able to map raw data to complex high semantic level outputs, such as in the case of automatic image description. This ability is enabling us to tackle problems that are increasingly more complex and at a higher semantic level of abstraction, leading to the advancement of computer vision based solutions in diverse fields of application. At the same time, there is a growing interest in undestanding what these models are representing. For instance, although new supposedly better models are “launched” routinely, rarely it is clear why they are better than the previous models. In this project we are interested in undestanding what these models are representing and in gaining some control on their training and behavior. For that, we are working towards finding ways to enforce more explicit representations of structural information, and exploring visualization techniques. These studies and explorations are done in the context of different applications, including astronomical images, plankton images, document images, natural scene images, and much more.
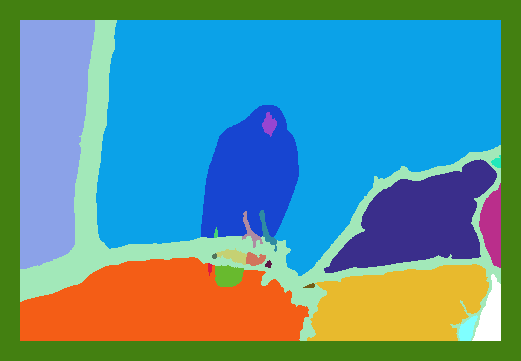
- Pattern recognition
- Machine learning
- Deep Learning
- Images (classification, segmentation)
- Object detection, image description
- Handwriting recognition
- Explainability
Department of Computer Science
Institute of Mathematics and Statistics
University of São Paulo
Project supported by FAPESP